Use Case: Robust Object Segmentation with SAM (Segment-Anything Model)¶
In this tutorial, we show how to wrap a SAM model with Sample
wrapper to output the uncertainty in masks.
The Segment Anything Model (SAM) is a tool for precise image segmentation, it produces high quality object masks from input prompts such as points or boxes. It can be used to generate masks for objects in an image. In this tutorial, we load a trained SAM model and wrap it with sample
wrapper.
Step1: Initial Setup¶
Install SAM model and download weights¶
Install SAM with the following commend:
pip install git+https://github.com/facebookresearch/segment-anything.git
More details about the installation can be found here: https://github.com/facebookresearch/segment-anything/tree/main
Download trained weights of SAM model
!wget -nc https://dl.fbaipublicfiles.com/segment_anything/sam_vit_h_4b8939.pth
--2024-05-22 22:38:36-- https://dl.fbaipublicfiles.com/segment_anything/sam_vit_h_4b8939.pth
Resolving dl.fbaipublicfiles.com (dl.fbaipublicfiles.com)...
13.249.190.102, 13.249.190.9, 13.249.190.74, ...
Connecting to dl.fbaipublicfiles.com (dl.fbaipublicfiles.com)|13.249.190.102|:443... connected.
HTTP request sent, awaiting response... 200 OK
Length: 2564550879 (2.4G) [binary/octet-stream]
Saving to: ‘sam_vit_h_4b8939.pth’
sam_vit_h_4b8939.pt 100%[===================>] 2.39G 103MB/s in 23s
2024-05-22 22:38:59 (107 MB/s) - ‘sam_vit_h_4b8939.pth’ saved [2564550879/2564550879]
Import requirements¶
from segment_anything import sam_model_registry
from segment_anything.utils.transforms import ResizeLongestSide
import torch
from torch import nn
from torch.nn import functional as F
from typing import Optional, Tuple
import matplotlib.pyplot as plt
from matplotlib.cm import ScalarMappable, jet # type: ignore
from matplotlib.colors import Normalize
import numpy as np
import cv2
Initialize Sam model and load weights¶
# LOAD PARAMETERS SPECIFIC TO THE ORIGINAL TRAINING RUN. These should be constant unless we decide to do a separate training
image_size = 1024
original_weight_path = "sam_vit_h_4b8939.pth"
device = torch.device("cuda")
sam = sam_model_registry["vit_h"](checkpoint=original_weight_path).to(device).eval()
Prompt Encoder (Preprocessor)¶
PreProcessor
is a class that implements some required pre-processing steps before we give the prompt (selected coordinates in this case) to the prompt encoder. These preprocessing steps (functions) are taken directly from the segment-anything library.
We pass the prompt encoder module to the PreProcessor()
so that we can call the PreProcessor()
directly with the coordinates to get the prompt embeddings.
class PreProcessor(nn.Module):
def __init__(self, prompt_encoder, device):
super().__init__()
self.device = device
self.image_size = 1024
pixel_mean = [123.675, 116.28, 103.53]
pixel_std = [58.395, 57.12, 57.375]
self.register_buffer(
"pixel_mean", torch.tensor(pixel_mean, device=device).view(-1, 1, 1), False
)
self.register_buffer(
"pixel_std", torch.tensor(pixel_std, device=device).view(-1, 1, 1), False
)
self.prompt_encoder = prompt_encoder
self.transform = ResizeLongestSide(self.image_size)
def __call__(self, point_coords, point_labels, image):
input_image = self.set_image(image=image)
sparse_embeddings, dense_embeddings = self.set_prompt(
point_coords=point_coords, point_labels=point_labels
)
return input_image, sparse_embeddings, dense_embeddings
def set_prompt(
self,
point_coords: Optional[np.ndarray] = None,
point_labels: Optional[np.ndarray] = None,
box: Optional[np.ndarray] = None,
mask_input: Optional[np.ndarray] = None,
multimask_output: bool = True,
return_logits: bool = False,
) -> Tuple[np.ndarray, np.ndarray]:
"""
Predict masks for the given input prompts, using the currently set image.
Arguments:
point_coords (np.ndarray or None): A Nx2 array of point prompts to the
model. Each point is in (X,Y) in pixels.
point_labels (np.ndarray or None): A length N array of labels for the
point prompts. 1 indicates a foreground point and 0 indicates a
background point.
box (np.ndarray or None): A length 4 array given a box prompt to the
model, in XYXY format.
mask_input (np.ndarray): A low resolution mask input to the model, typically
coming from a previous prediction iteration. Has form 1xHxW, where
for SAM, H=W=256.
multimask_output (bool): If true, the model will return three masks.
For ambiguous input prompts (such as a single click), this will often
produce better masks than a single prediction. If only a single
mask is needed, the model's predicted quality score can be used
to select the best mask. For non-ambiguous prompts, such as multiple
input prompts, multimask_output=False can give better results.
return_logits (bool): If true, returns un-thresholded masks logits
instead of a binary mask.
Returns:
(np.ndarray): The output masks in CxHxW format, where C is the
number of masks, and (H, W) is the original image size.
(np.ndarray): An array of length C containing the model's
predictions for the quality of each mask.
(np.ndarray): An array of shape CxHxW, where C is the number
of masks and H=W=256. These low resolution logits can be passed to
a subsequent iteration as mask input.
"""
# Transform input prompts
coords_torch, labels_torch, box_torch, mask_input_torch = None, None, None, None
if point_coords is not None:
assert (
point_labels is not None
), "point_labels must be supplied if point_coords is supplied."
point_coords = self.transform.apply_coords(point_coords, self.original_size)
coords_torch = torch.as_tensor(
point_coords, dtype=torch.float, device=self.device
)
labels_torch = torch.as_tensor(
point_labels, dtype=torch.int, device=self.device
)
coords_torch, labels_torch = coords_torch[None, :, :], labels_torch[None, :]
if box is not None:
box = self.transform.apply_boxes(box, self.original_size)
box_torch = torch.as_tensor(box, dtype=torch.float, device=self.device)
box_torch = box_torch[None, :]
if mask_input is not None:
mask_input_torch = torch.as_tensor(
mask_input, dtype=torch.float, device=self.device
)
mask_input_torch = mask_input_torch[None, :, :, :]
if coords_torch is not None:
points = (coords_torch, labels_torch)
else:
points = None
# Embed prompts
sparse_embeddings, dense_embeddings = self.prompt_encoder(
points=points,
boxes=box_torch,
masks=mask_input_torch,
)
return sparse_embeddings, dense_embeddings
def set_image(
self,
image: np.ndarray,
image_format: str = "RGB",
) -> torch.Tensor:
"""
Calculates the image embeddings for the provided image, allowing
masks to be predicted with the 'predict' method.
Arguments:
image (np.ndarray): The image for calculating masks. Expects an
image in HWC uint8 format, with pixel values in [0, 255].
image_format (str): The color format of the image, in ['RGB', 'BGR'].
"""
assert image_format in [
"RGB",
"BGR",
], f"image_format must be in ['RGB', 'BGR'], is {image_format}."
# if image_format != self.model.image_format:
# image = image[..., ::-1]
# Transform the image to the form expected by the model
input_image = self.transform.apply_image(image)
input_image_torch = torch.as_tensor(input_image, device=self.device)
input_image_torch = input_image_torch.permute(2, 0, 1).contiguous()[
None, :, :, :
]
input_image = self.set_torch_image(input_image_torch, image.shape[:2])
return input_image
@torch.no_grad()
def set_torch_image(
self,
transformed_image: torch.Tensor,
original_image_size: Tuple[int, ...],
) -> torch.Tensor:
"""
Calculates the image embeddings for the provided image, allowing
masks to be predicted with the 'predict' method. Expects the input
image to be already transformed to the format expected by the model.
Arguments:
transformed_image (torch.Tensor): The input image, with shape
1x3xHxW, which has been transformed with ResizeLongestSide.
original_image_size (tuple(int, int)): The size of the image
before transformation, in (H, W) format.
"""
assert (
len(transformed_image.shape) == 4
and transformed_image.shape[1] == 3
and max(*transformed_image.shape[2:]) == self.image_size
), f"set_torch_image input must be BCHW with long side {self.image_size}."
# self.reset_image()
self.original_size = original_image_size
self.input_size = tuple(transformed_image.shape[-2:])
return self.preprocess(transformed_image)
def preprocess(self, x: torch.Tensor) -> torch.Tensor:
"""Normalize pixel values and pad to a square input."""
# Normalize colors
x = (x - self.pixel_mean) / self.pixel_std
# Pad
h, w = x.shape[-2:]
padh = self.image_size - h
padw = self.image_size - w
x = F.pad(x, (0, padw, 0, padh))
return x
preprocessor = PreProcessor(prompt_encoder=sam.prompt_encoder, device=device)
Postprocessing¶
The two functions below resize_longest_image_size()
and mask_postprocessing()
are post-processing functions also taken directly from segment_anything library. Mask Decoder
always returns the same shape. So these functions are used to stretch the predicted masks to match with the original input shape.
def resize_longest_image_size(input_image_size: torch.Tensor, longest_side: int):
input_image_size = input_image_size.to(torch.float32)
scale = longest_side / torch.max(input_image_size)
transformed_size = scale * input_image_size
transformed_size = torch.floor(transformed_size + 0.5).to(torch.int64)
return transformed_size
def mask_postprocessing(
masks: torch.Tensor, orig_im_size: torch.Tensor
) -> torch.Tensor:
masks = F.interpolate(
masks,
size=(image_size, image_size),
mode="bilinear",
align_corners=False,
)
prepadded_size = resize_longest_image_size(orig_im_size, image_size).to(torch.int64)
masks = masks[..., : prepadded_size[0], : prepadded_size[1]] # type: ignore
orig_im_size = orig_im_size.to(torch.int64)
h, w = orig_im_size[0], orig_im_size[1]
masks = F.interpolate(masks, size=(h, w), mode="bilinear", align_corners=False)
return masks
Step 2: Wrap with capsa-torch¶
In this example, we are wrapping only the MaskDecoder
module. However, we could also try wrapping PromptEncoder
, ImageEncoder
, or even the entire SAM model all together. All these different approaches yield different results in terms of meaning and interpretability of risk.
Here we use the Sample Wrapper
which yields epistemic uncertainty. We could also try wrapping with Vote Wrapper
or Sculpt Wrapper
. Vote Wrapper
should yield similar results to Sample Wrapper
after further training. However, the risk values obtained by wrapping with Sculpt Wrapper
and further training will have different meanings since the Sculpt Wrapper
estimate aleatoric uncertainty.
from capsa_torch import sample
# Initialize wrapper
drop_rate = 0.01 # This determines how noisy the model is after wrapping
n_samples = 10 # Number of times to sample from stochastic model
wrapper = sample.Wrapper(n_samples=n_samples, distribution=sample.Bernoulli(drop_rate), symbolic_trace=False)
# Wrap mask decoder by calling it with the wrapper and move to device
wrapped_mask_decoder = wrapper(sam.mask_decoder).to(device)
Predict¶
We provide an image for object masking, the user can also use their own figure for testing, simply change the IMAGE_PATH
.
IMAGE_PATH = "IMG_1081.jpg"
# Coordinates of the selected points in the image
COORDINATES = np.array([[2000, 2000]])
# Labels for the selected points in the image. 1 says the associated point is part of the object, while 0 says the opposite.
COORDINATE_LABELS = np.array([1])
image = cv2.cvtColor(cv2.imread(IMAGE_PATH), cv2.COLOR_BGR2RGB)
# Preprocess inputs with PreProcessor (Prompt Encoder)
input_image, sparse_embeddings, dense_embeddings = preprocessor(
point_coords=COORDINATES, point_labels=COORDINATE_LABELS, image=image
)
# Encode image to get embeddings
with torch.no_grad():
image_embeddings = sam.image_encoder(input_image)
# Pass the image and prompt embeddings to wrapped mask decoder to get the low resolution mask, and it's risk
with torch.no_grad():
(low_res_mask, iou_prediction),(low_res_mask_risk, iou_prediction_risk) = wrapped_mask_decoder(
return_risk=True,
image_embeddings=image_embeddings,
image_pe=sam.prompt_encoder.get_dense_pe(),
sparse_prompt_embeddings=sparse_embeddings,
dense_prompt_embeddings=dense_embeddings,
multimask_output=True,
)
# We then pass these two arrays to the post-processing function to upscale them to match the original image's size
original_size = torch.Tensor(preprocessor.original_size).clone().detach()
upscaled_mask = mask_postprocessing(low_res_mask, original_size)
upscaled_mask_risk = mask_postprocessing(low_res_mask_risk, original_size)
With multimask_output=True
, SAM outputs 3 masks in low_res_mask
, together with scores in their model’s own estimation of the quality of these masks in iou_prediction
. Our wrapper predicts the risk for both, saving as low_res_mask_risk
and iou_prediction_risk
.
Compute p-values¶
Mask decoder outputs an array with values between -infinity and +infinity. If a pixel’s value is bigger than 0
, then this means that particular pixel is part of the mask. Another way to say this is that the decision threshold is 0
.
We can treat the output of our capsa-torch wrapped model as a normal distribution where the mean is the upscaled_mask, and the scale (standard deviation) is the risk. If we do this, individual pixels represent independent normal distributions.
With this intuition, we can then determine the model’s confidence in it’s predictions. We do this by using the CDF of the Normal distribution to compute the probability that our sampled mean value is on the correct side of the 0
threshold.
dist = torch.distributions.Normal(loc=upscaled_mask, scale=upscaled_mask_risk)
# P(true mean > 0)
p_value_greater_than_0 = 1 - dist.cdf(torch.zeros_like(upscaled_mask))
p_value_wrong = p_value_greater_than_0.clone()
p_value_wrong[upscaled_mask < 0] = 1 - p_value_greater_than_0[upscaled_mask < 0] # if sampled mean < 0, p=P(true mean < 0)
p_value_greater_than_0 = p_value_greater_than_0.cpu().numpy()[0]
p_value_wrong = p_value_wrong.cpu().numpy()[0]
quanlity_of_mask = iou_prediction.cpu().numpy()[0]
Step 3: Testing & Evaluation¶
Define helper functions¶
Functions below are helper functions that can add masks (show_mask()
), points (show_points()
), or risks (show_std()
) to a given matplotlib figure.
def show_mask(mask, ax):
color = np.array([30 / 255, 144 / 255, 255 / 255, 0.6])
h, w = mask.shape[-2:]
mask_image = mask.reshape(h, w, 1) * color.reshape(1, 1, -1)
ax.imshow(mask_image)
def show_points(coords, labels, ax, marker_size=100):
pos_points = coords[labels == 1]
neg_points = coords[labels == 0]
ax.scatter(
pos_points[:, 0],
pos_points[:, 1],
color="green",
marker="*",
s=marker_size,
edgecolor="white",
linewidth=1.25,
)
ax.scatter(
neg_points[:, 0],
neg_points[:, 1],
color="red",
marker="*",
s=marker_size,
edgecolor="white",
linewidth=1.25,
)
def show_std(mask_std, ax, colorbar_ax, ignore_outliers=False):
# HEATMAP
vmin = np.percentile(mask_std, 2) if ignore_outliers else mask_std.min()
vmax = np.percentile(mask_std, 98) if ignore_outliers else mask_std.max()
norm = Normalize(vmin=vmin, vmax=vmax)
rgba_values = jet(norm(mask_std))
ax.imshow(rgba_values)
ax.axis("off")
plt.colorbar(ScalarMappable(norm=norm, cmap="jet"), cax=colorbar_ax)
Visualize the mask and risk¶
We visualize the masks and risks for the three masks with SAM model’s own estimation of qualities of masks.
import matplotlib.pyplot as plt
def plot_each_mask(index, image, coordinates, coordinate_labels, p_value_greater_than_0, p_value_wrong, quality_of_mask):
# Create an axis for the colorbar
fig, ax = plt.subplots(nrows=1, ncols=3, figsize=(15, 5))
colorbar_ax = fig.add_axes([0.92, 0.1, 0.02, 0.8])
# Ax 0: Image w/ marker
ax[0].axis("off")
ax[0].imshow(image)
show_points(coordinates, coordinate_labels, ax[0])
ax[0].title.set_text("Image w/ Selection marker")
# Ax 1: Image w/ mask + marker
ax[1].axis("off")
ax[1].imshow(image)
show_mask(p_value_greater_than_0[index], ax[1])
show_points(coordinates, coordinate_labels, ax[1])
ax[1].title.set_text("Image w/ Predicted mask")
# Ax 2: p-value of each pixel prediction being incorrect
show_points(coordinates, coordinate_labels, ax[2])
show_std(p_value_wrong[index], ax[2], colorbar_ax)
ax[2].title.set_text("Prediction confidence")
# Adjust the layout to make space for the title at the bottom
fig.subplots_adjust(wspace=0, hspace=0.3, bottom=0.15)
# Add the title at the bottom
fig.suptitle(f"Figure {index + 1}: SAM quality of mask: {quality_of_mask[index]:.2f}", fontsize=16, y=0.05)
plt.show()
# Assuming image, COORDINATES, COORDINATE_LABELS, p_value_greater_than_0, p_value_wrong, and quality_of_mask are defined
for i in range(3):
plot_each_mask(i, image, COORDINATES, COORDINATE_LABELS, p_value_greater_than_0, p_value_wrong, quanlity_of_mask)
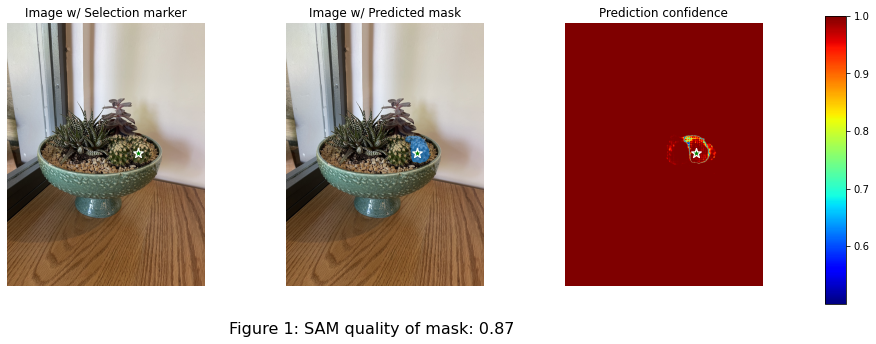
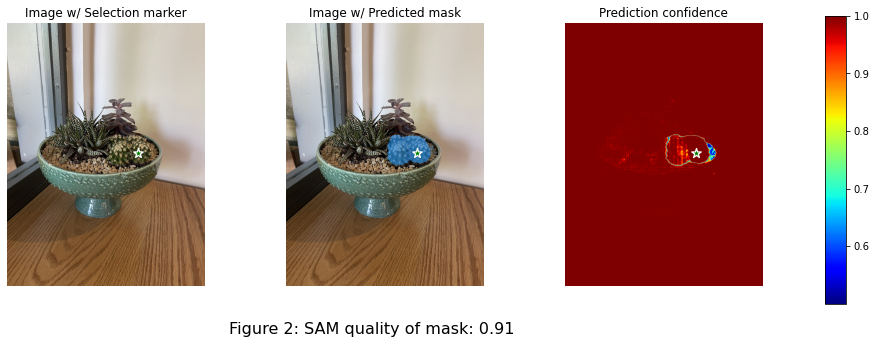
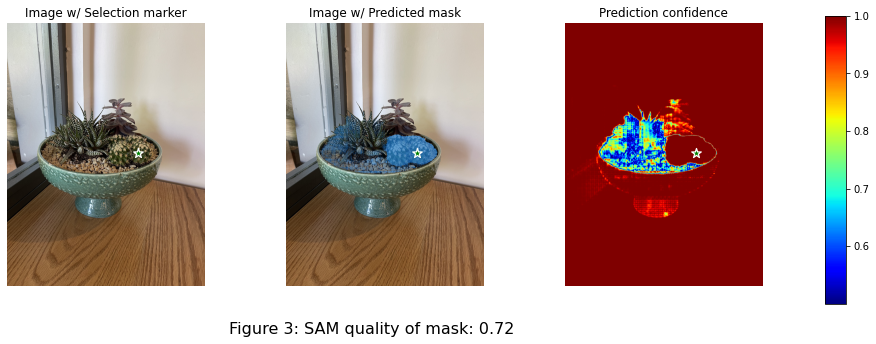
The prediction confidence shows the confidence of object masks predicted by the SAM model for the input point. The edges and fine structures of the selected object are expected to have a higher risk, indicated by the lower value on the colorbar. We can also see that the mask with lower quanlity score generally has more area with high risks, while the SAM model only predicts a number estimating the quality of their mask, our wrapped model can estimate the distribution of the risk over the image.